Correlation analysis can analyze which products or product combinations are often purchased together. If you find a combination of these products that are often purchased together, you can do a lot of valuable things. These values can be embodied in at least the following three aspects:
1. Guide the arrangement and placement of products in offline stores
Commodities that are often purchased together can be placed close to the space in the offline store, which is convenient for customers to choose and reduces the time for customers to find. This improves the user experience and intangibly increases the sales volume of the goods. Get more sales revenue.
If it is an online store like Taobao and JD.com, one of the frequently purchased products can be used as a related recommendation for another product, which increases the probability that a combination of related products will be exposed together and promotes user purchase conversion (the picture below is in the Hema APP Browse Baxi, the matching recommendations given below). We will explain this part in the third application scenario.
2. Optimize offline procurement, supply chain and inventory
Commodities that are often purchased together can be put together for overall planning in procurement, packaging, transportation, and inventory, such as selecting suppliers in the same region and storing them in similar places in the warehouse. For the introduction of this section, readers can search for related materials.
3. Provide data support for event marketing
Commodities that are often bought together can also be used for marketing activities (even the brand side of related commodities can also do joint marketing together), such as discounts when buying together, and buying one of the expensive ones to get the other cheaper ones that are often bought together, etc. Wait.
The above are just a few business cases that everyone can easily think of in the shopping basket correlation analysis. More application scenarios need to be tried and explored in combination with the actual situation of the enterprise. The most important thing in shopping basket analysis is actually to digitize the user's buying behavior. With data as the foundation and resources, what is left is to use data analysis, data mining, machine learning and other algorithms to explore the data and tap the potential commercial value. With user shopping behavior data, regardless of the commercial value obtained by correlation analysis, a lot of other commercial values can be unearthed. Below I will also do some simple sorting of other values of user shopping data, to provide you with some thinking ideas and a broader perspective.
As long as the shopping data of customers is collected for a period of time, and the data is digitized (entered into the information system of the supermarket), simple statistical analysis can be used (if the data is stored in a relational database such as SQL, only one SQL statement is required). Count out which items are frequently purchased by customers.
What is the use of knowing the most frequently purchased products (ie best-selling or daily-selling products)? I believe you can easily think of the usefulness. First of all, it is definitely helpful for shopping malls to purchase and order. The source of the most frequently purchased commodities and supply chain need to be well protected, and the shopping malls need to pay attention to ensure sufficient supply. In addition, the placement of hot-purchased goods can also be optimized. Many stores place popular items in the most conspicuous and most accessible places for customers. Many supermarkets put chewing gum and condoms at the checkout place for this reason.
The purchase of many commodities may have time period and seasonal fluctuations. For example, fresh milk is usually purchased in the morning, while mosquito coils are purchased in summer. Through the analysis of the time of purchase, we can roughly know the fluctuation law of certain commodities in time and season, and analyze the law of the above purchase time cycle. Better help businesses purchase, reserve, and market goods.
If combined with user portrait information (there are many ways to obtain user portrait information, for example, some companies can have a membership system, and users can register for an online APP, so that user related information can be obtained. In addition, if there are cameras and other equipment in the mall, it is also possible If you get a general portrait of the user, these are beyond the scope of this article and will not be repeated), you can analyze the purchase characteristics of different groups (such as the purchase differences of different genders, different age groups, different incomes, etc.), and do personalized marketing.
Many retail companies are chain companies (or even multinational companies). They can collect sales data from different stores, analyze geographically related data in different regions, explore the purchasing behavior of users in different regions, and make customized strategies for different regions. . For example, users in different regions may have different hot-selling products, users in different regions often buy different products together, and seasonal changes in the purchase period of users in different regions may also be different. This more refined analysis is conducive to adopting local strategies for different regions (even different stores).
Many retail companies have both wired online APP and offline physical stores (such as Hema has Hema APP and Hema offline stores), so how to link online and offline is very valuable. Online stores also have so-called shopping baskets (the products purchased by the user in the app can also be regarded as a shopping basket), which can also use the methods and strategies of shopping basket analysis.
If a retail company only starts online, and then starts offline, then online data analysis can empower offline business. For example, based on online user purchase behavior data analysis, you can also dig out the aforementioned various information (such as popular products, products that are often purchased together, etc.), and this information can be directly used for the selection and arrangement of offline stores . vice versa.
Online data can also be used for location selection of offline stores. For example, it is possible to analyze the purchase situation of online users and obtain the distribution of users in different regions, which is helpful to guide the number decision and location selection of stores to open stores in different regions.
In short, in the context of the current Internet dividends being exhausted, online and offline integration and linkage are the direction and opportunity. Online and offline integration and mutual empowerment are the future trends of the retail industry. Whoever can grasp this trend will get the ticket to the next golden development stage of the retail industry (even any industry).
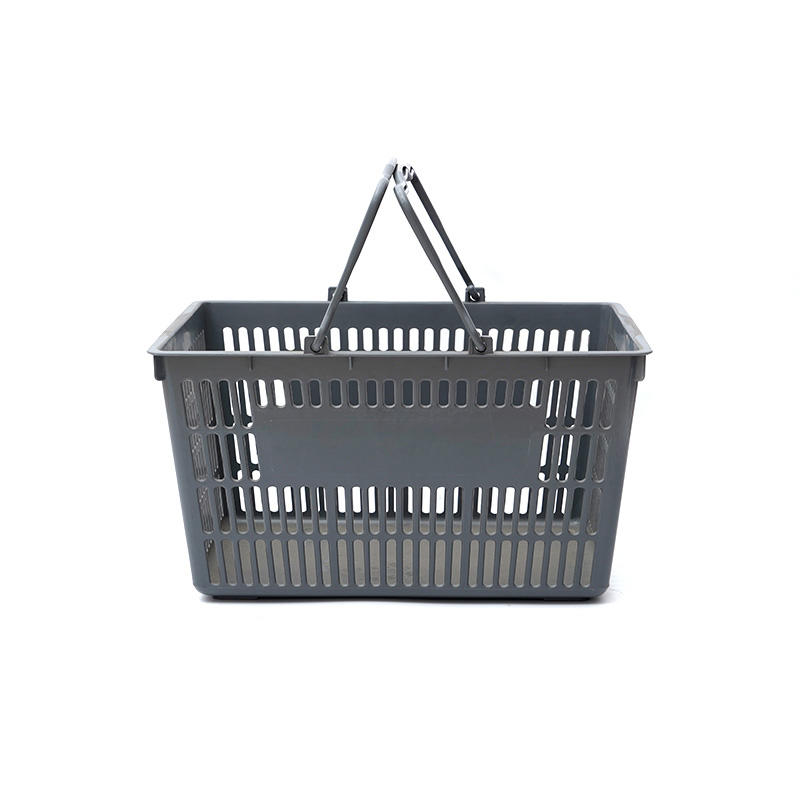